Gaining Perspective
On 11 September 2019, Andrew successfully passed the defense of his PhD dissertation, entitled “Preference Learning and Similarity Learning Perspectives on Personalized Recommendation”.
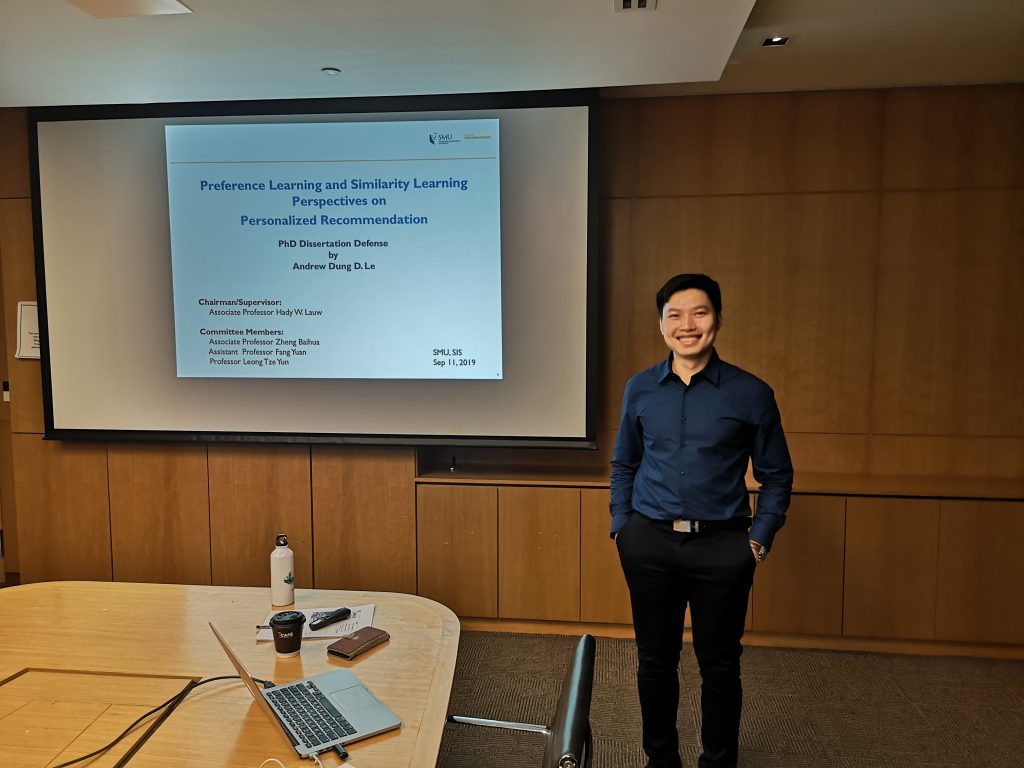
He posits that there are different modes of recommendation. In one scenario, the objective is to assess the preference of a user for an item. In another scenario, the objective is help a user who is interested in a particular item to identify other similar items to consider.
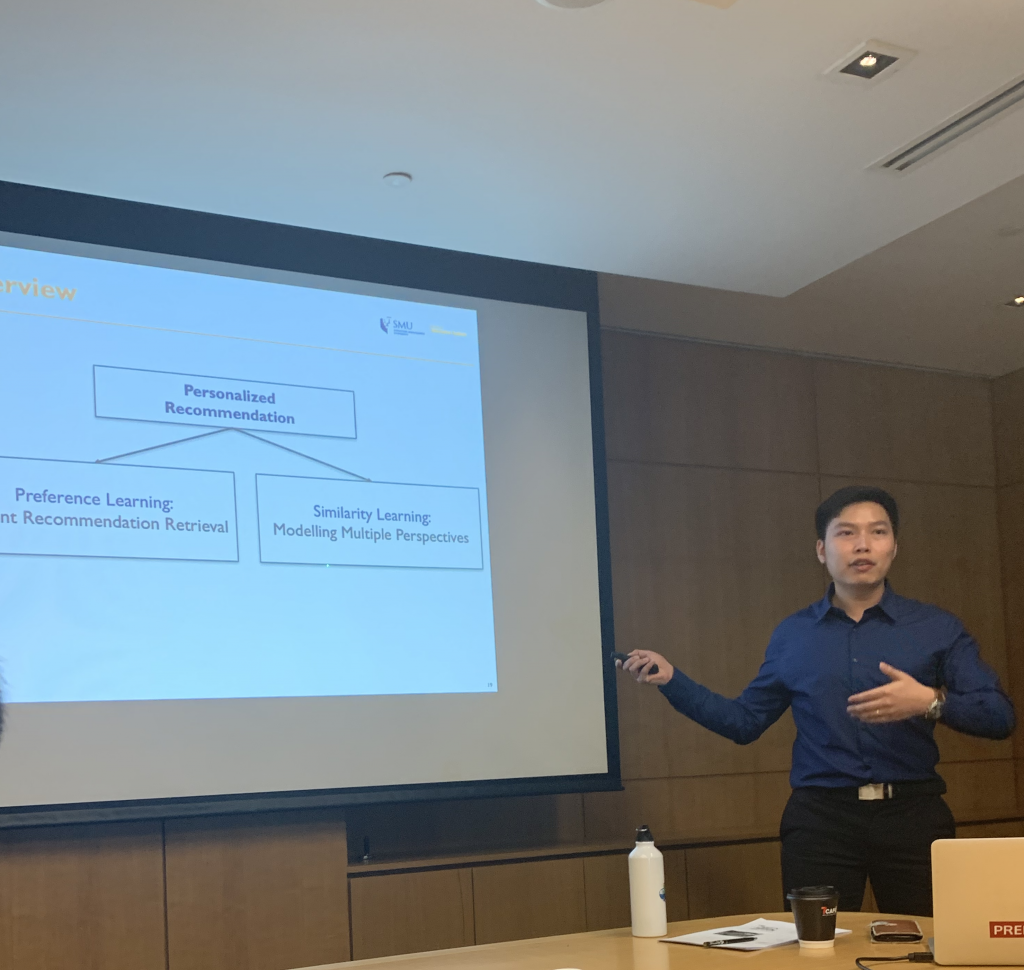
Within the preference learning perspective, his focus is on the retrieval efficiency of recommendation. While recommendation models could be updated offline, the top-K personalized recommendations often need to be retrieved online. By designing indexable models in the first place, we can improve the compatibility of the latent factors with the underlying index structures used to retrieve preferred items in real time.
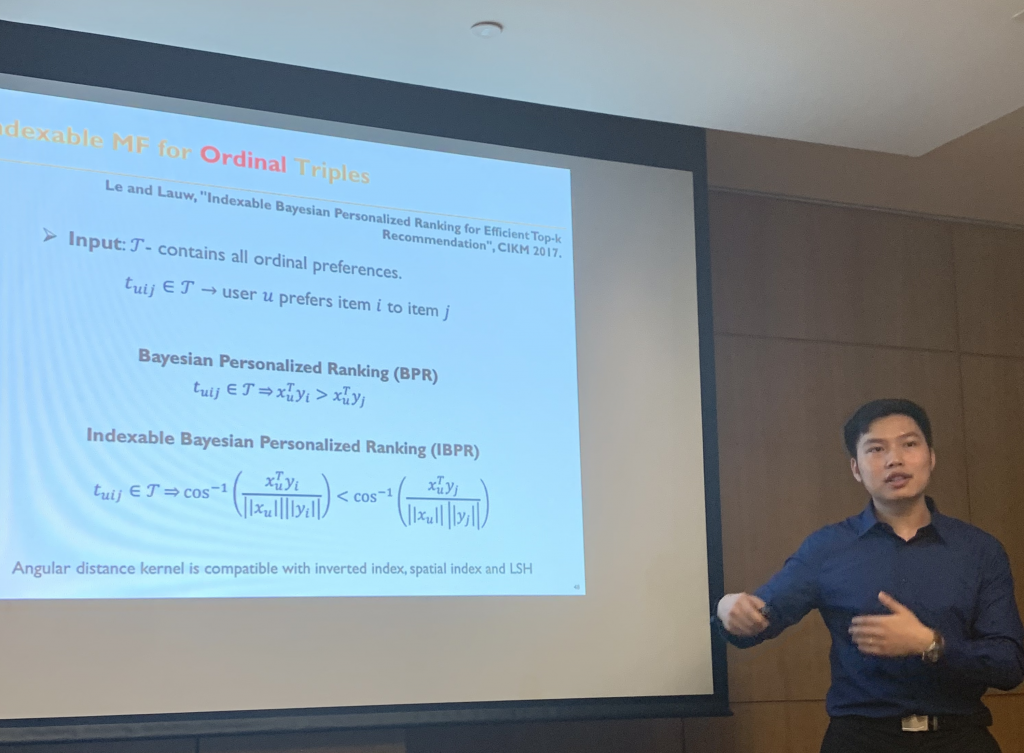
Within the similarity learning perspective, he develops the intuition that two items may be similar to one user, but may be different to another user. Hence, there is a need for similarity learning models that can differentiate these viewpoints, yet also allowing them to collaborate in sharing information where there is a consensus.
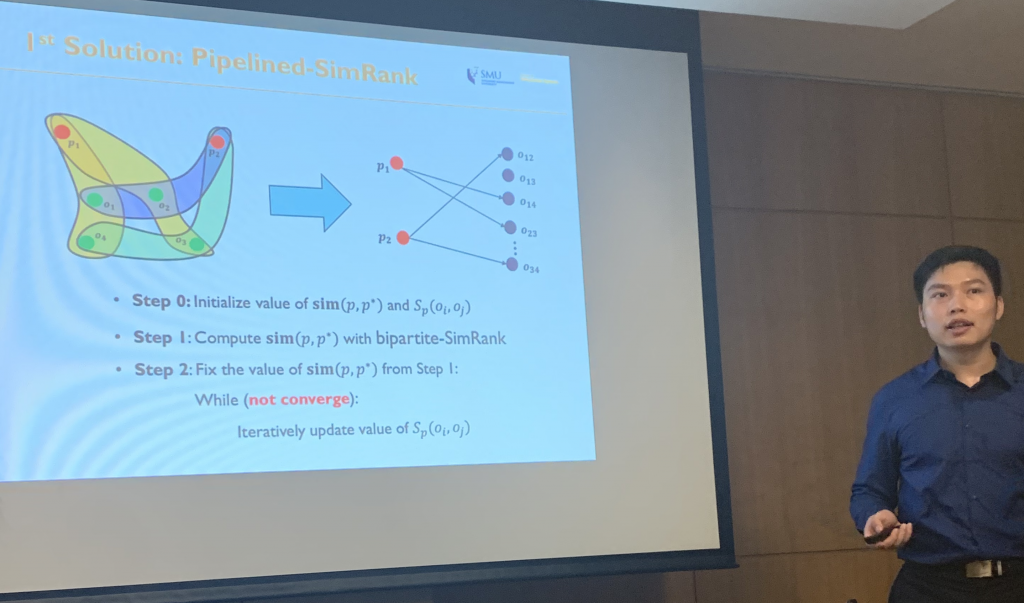
In the five years he has been with us, at first as a research engineer then as a PhD candidate, Andrew has garnered various accomplishments, including winning SMU Presidential Doctoral Fellowships as well as PhD Student Life Awards, in addition to attaining publications in reputable data mining and AI conferences.
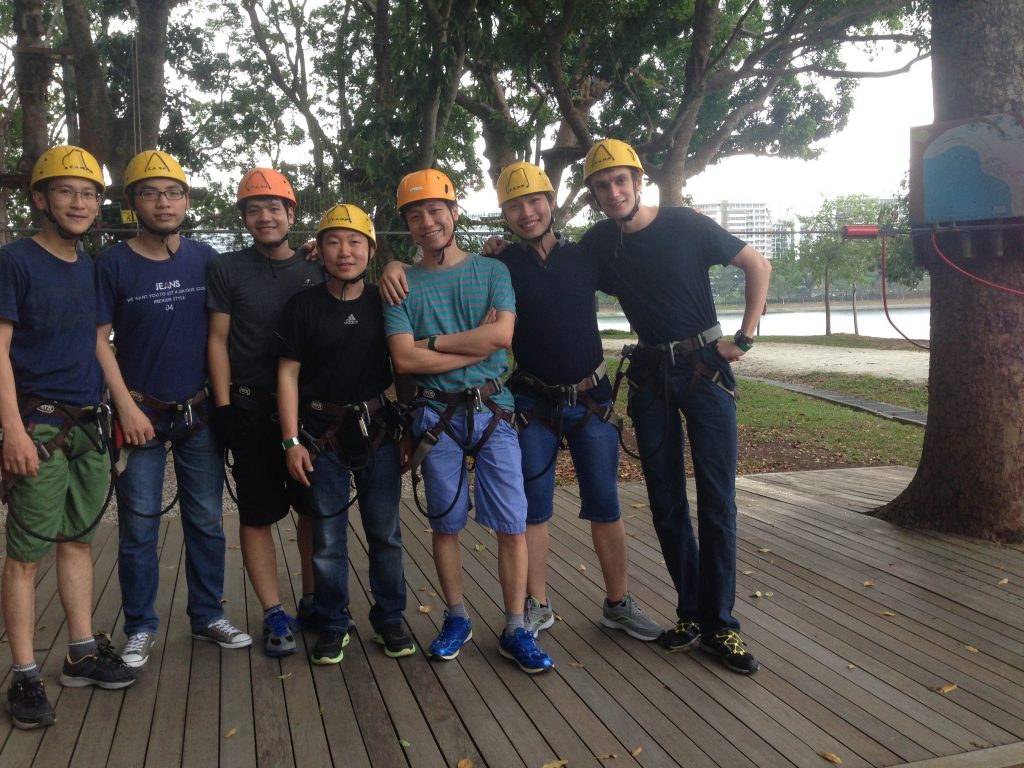
(from left: Tuan Le, Trong Nguyen, Trong Le, Hady, Son, Andrew, Maksim)
In celebratory mood, we headed down to OverEasy at Fullerton. The mojitos (his favourite drink) went down easily (perhaps overly so). That probably helped us to gain some perspective in appreciating the achievement that was completing one’s PhD. Surely, one is glad it’s over; we all know it ain’t easy.
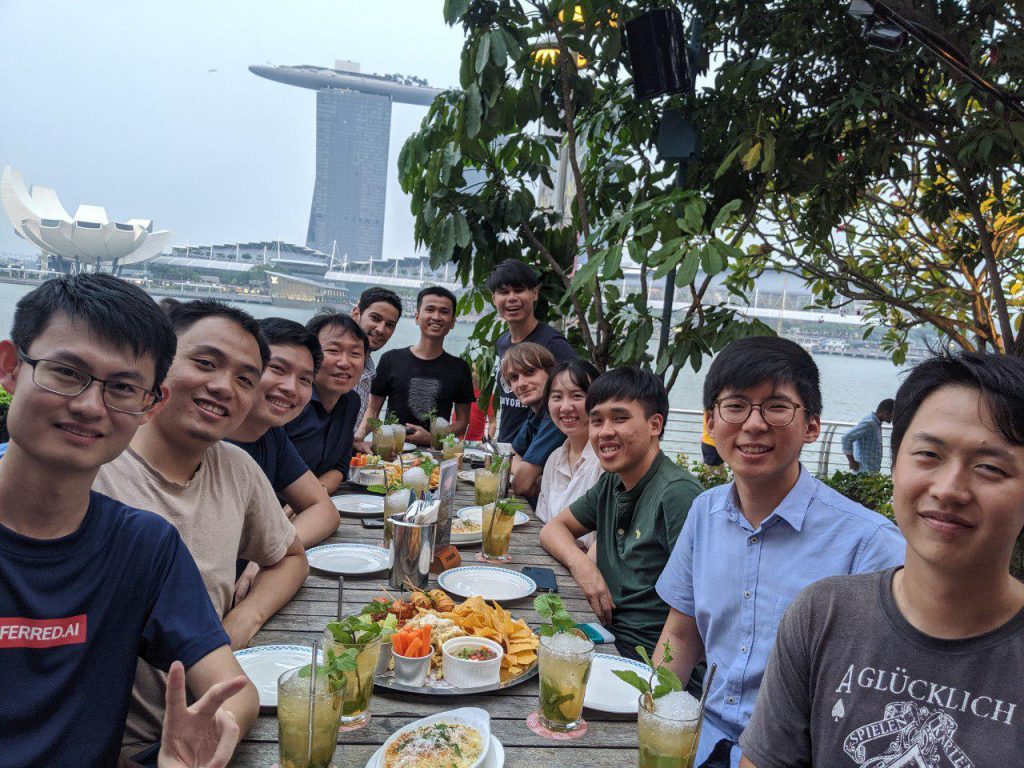
Congratulations Andrew on a PhD well-earned!